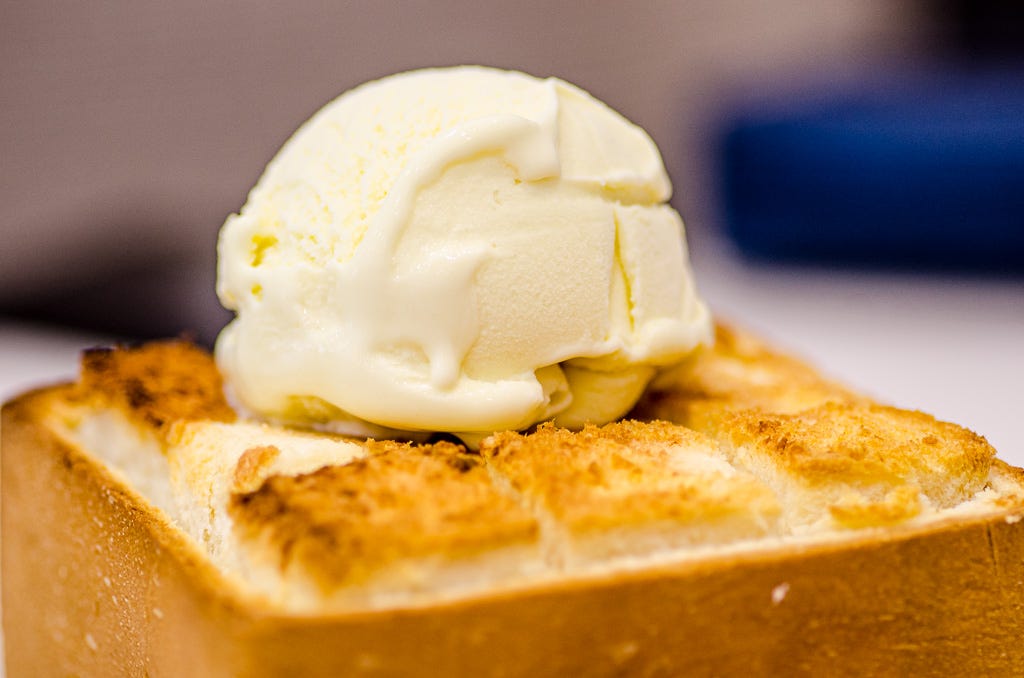
Earlier this year, a bunch of data folk started making bemused, and sometimes snarky comments about a steady trickle of news about streaming subscription services struggling with “churn” as if it were some new concept.
Now, I think few people are willing to believe that people at the likes of HBO and Disney have never heard of the concept of subscriptions and churn before. That concept isn’t rocket science. But the press seemed to like to frame it that way for some reason. Maybe it’s because the writers wanted to emphasize that these big media companies were struggling with a new business model, while at the same time needing to express the struggle to a naïve audience? I dunno.
Either way, I’ve no doubt that while the big media companies know OF churn and subscriptions, they’re very likely struggling to grasp and understand it. Because just about everyone else who has a business that relies on repeat customers has trouble with churn. Because churn is difficult.
If anything, I believe successful examples of dealing with churn are the vast minority of instances. I’ve personally went on churn-hunting efforts multiple times in my career, and my hit rate has been pretty abysmal.
That’s why this following tweet caught my attention, as well as the attention of a lot of data folk. It’s very much worth your time to read the thread.

So, what’s with churn? Why’s it so difficult that everyone who’s every worked on the problem immediately perks up and pays attention when someone puts an example up?
Churn can be hard to even define
At a super high level, churn is “when someone who was paying you stops”. It’s a very simple concept, but gets thorny down in the details.
Subscription-type services, like the streaming services mentioned above, have it the easiest. There’s a pretty clear event for when people stop paying you — they cancel their subscription in some way. Usually they say they don’t want to renew and their contract eventually expires. There could be other complexities that make pinpointing exactly “when” churn happened more difficult, like grace periods, non-payment, etc. Was it when they hit the cancel button, or when the subscription finally lapses, or after their X day non-payment grace period? But at the least there’s an actual logged event. I’ll just label them as positive-type churn businesses.
Meanwhile, there are lots and lots of businesses where people don’t have a subscription relationship, but can go from “paying you regularly” to “not”. But there’s no positive, easily recordable event that marks that event. Common businesses that do this are most forms of e-commerce services, and free services where user value is in repeated activity and usage.
In these negative-type churn situations, defining churn is a challenge. You have to figure out a point where you think a customer has switched from “is just waiting between purchases/usages” to “has decided not to purchase any more”. Obviously there’s uncertainty involved in this since different people will have different needs and behavior patterns. It’s pretty easy to accidentally declare a regular daily user has churned when they instead just went on vacation for a week.
The research needed to even make a good heuristic for “this customer has churned” can be pretty significant, especially if the customer base has a large number of different behavior patterns mixed together. Many first-time churn projects find themselves trying to address all these issues and never get far beyond even this step. Don’t worry though, this is normal. This work is hard!
Churn is often far removed from its decision
Just defining churn isn’t enough to do anything about it. Usually, by the time a customer has actively decided to hit a button to stop paying you, they’ve been thinking about it for a while already. Think of that subscription that you haven’t used in over a year but haven’t gotten around to cancelling because it’s only a few bucks. Trying to change a customer who has decided to churn already is very difficult.
Most methods of stopping churn, like giving discounts in an effort to convince someone to stay, can be pretty ineffective. It’s similar to sticking “50% off” stickers on a burning car wreck. The users are long gone and only a tiny fraction can be enticed to even read your offer emails at this point.
Other forms of churn prevention can be downright sketchy, unethical, or maybe even illegal. Think of all the cancellation processes that require a long and difficult process to cancel.
Above is timely tweet on the night of publishing this, here’s a tweet about part of Adobe’s anti-churn strategy… a giant red warning that bills you AN EXTRA penalty amount on top of your monthly subscription fee, “justified” because the agreement terms are yearly, but paid monthly. Plenty of people are rightly raging over this anti-consumer behavior.
A much more effective, but difficult strategy, to improve churn is to catch people who are considering churning for some reason, and find a way to convince them to continue being customers in a positive way.
Hopefully you can see the difficulty — churn decisions are in a user’s head. You can’t reach in and measure it. I’m not even sure if you can develop a survey that measures it without weird downstream effects. The decision also can happen long before the churn actually happens. I know I’ve debated on cancelling a service for months before researching and testing an alternative before actually leaving.
Churn analysis requires deep, high quality data collection
So if we commit ourselves to the theory that we can predict churn before the decision has been finalized, we need to figure out what goes into that prediction. We assume that somewhere in the user’s historic behavior and interactions with our product, we can see signs of things going wrong, signs that the customer is becoming increasingly unhappy with our product. Then we want to intervene and convince them to stay.
What are those signs?
It’s often very hard to say. There are millions of possibilities that swirl in the mind that could potentially be a sign of potential churn, but you have to carefully figure out if they’re actually predictors of churn or not. But out of all those hypothesized predictors, what’s actually be tracked and measured in your systems? Is that data available for use and collected consistently for the months and years needed to run the analyses needed to figure out if they’re predictive or not?
Churn analysis relies upon the existence of rich longitudinal data. You can think of it as a late stage result of a long journey of instrumenting and measuring product usage and user behavior. This presents a bit of a dilemma for many businesses that are just starting up in their data collection and analysis processes. They want to answer this huge revenue driver question, but aren’t in a position to actually figure it out yet.
Churn is a data fishing expedition
Even when you’re sitting on tons of carefully collected data, there’s no guarantee that you’ll find very strong predictors of churn. Even if you find predictors, a bunch will prove to be things that are too late in the decision making process for you to effectively reverse.
So, as in the example above, you’re going to have to meticulously sift through factor by factor, hitting lots of dead ends along the way. The hardest part of the process is that say you identify a promising factor. You then need to show people in product and engineering. They need to figure out how to make changes to make that part of the product better, and test it out. Months have passed. Only after lots of work does everyone discover that it doesn’t work very well. Then you go back to the drawing board and look for more factors.
To ramp up the difficulty even more, while initially you can rely on intuition to focus on areas most likely to affect churn, for example examining common support issues, outages, usage of major product features, at some point you’re going to run out of obvious candidates. Mature products have most of their rough edges already ground off, meaning there aren’t obvious wins to be had..
There’s no silver bullets, just grind
The distribution of causes of customer churn are very likely distributed along something that resembles a power law, so variants of the 80/20 rule usually apply. Usually, people take this to mean that they just have to find “the one thing” that’s causing all the churn. If only we could find that magic bullet, we’d solve churn, make millions, and go home.
Sadly, the reality is that the tail of the distribution tends to be pretty fat. Once you hunt down and defeat one big churn boss, another smaller one pops up to replace it. As the tweet w/ the successful churn mentions, there comes a point where it’s death by a thousand cuts.
Each of those cuts can be worth a lot of money, but you have to grind them out. Each is a little detective story, tracing through one or more customer’s histories, seeing what went wrong, and figuring out how to make things better in a generalizable way going forward.
Sometimes the solutions require a product fix or change. Sometimes the solution means adding a feature, or adding/fixing documentation. Other times it means having new protocols for support tickets or the support phone script. Other times it’s not obvious what the fix even should be. Very often, each one is completely unique and has little to do with anything else.
But it’s doable!
Just because something is a slog doesn’t mean it’s impossible. Improving churn is always possible, and you’ll be learning a huge amount of stuff along the way. Even if you don’t get to the end destination, you’ll definitely improve your data collection, improve how you think about and measure aspects of the business, and also find all sorts of unseen friction in things.
Definitely worth the journey.
About this newsletter
I’m Randy Au, currently a quantitative UX researcher, former data analyst, and general-purpose data and tech nerd. The Counting Stuff newsletter is a weekly data/tech blog about the less-than-sexy aspects about data science, UX research and tech. With occasional excursions into other fun topics.
All photos/drawings used are taken/created by Randy unless otherwise noted.
Supporting this newsletter:
This newsletter is free, share it with your friends guilt free! But if you like the content and want to apply pressure on me to write more, here’s some options:
Tweet me - Comments and questions are always welcome, they often inspire new posts
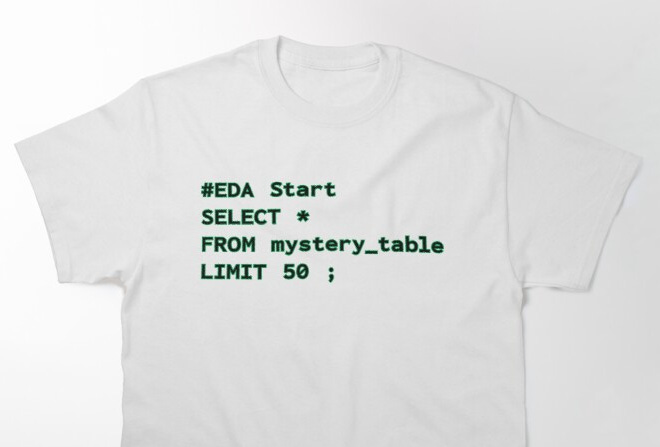