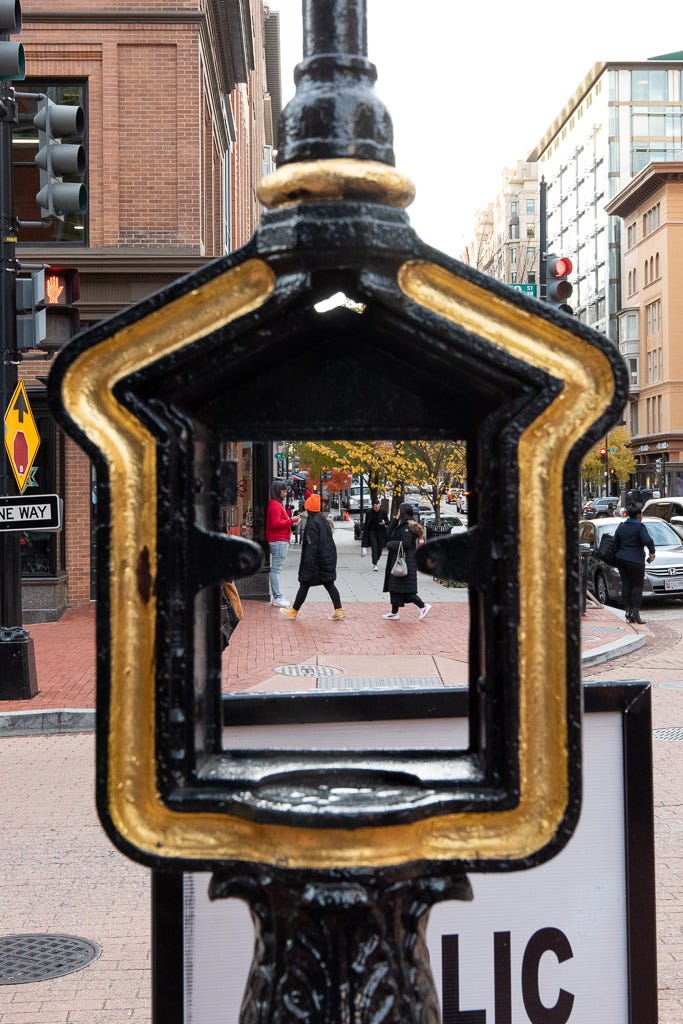
These days, while doing my best not touch my face, I’m watching the world at large take a crash course in basic data analysis. Since teaching data literacy is a big part of my job, I pay a bit more attention to people learning about data in my daily life. Yes, the way I’m coping with current events filled with increasing uncertainty and risk is to say “Look! This is a learning moment!”
I wish I knew who I can attribute this to, but I remember hearing something that proves true in these situations: Average people aren’t stupid, they’re just highly unmotivated to learn about things that seem irrelevant to them. But in times of crisis, like a cancer diagnosis [or looming pandemic], people can become experts VERY quickly.
I remember how when I had a personal medical issue that required a low-risk routine surgery. It took less than 24 hours after getting a diagnosis (a.k.a. a name to put into Google) before I had dove headfirst into medical papers describing prognoses, treatment methods and their risk assessments, etc. It’s easy to slog through mountains of information with the right motivation.
COVID-19 is providing much of the world with a huge motivation to learn about epidemiology and virology right now. While they’re probably not aware of it, these people are learning a lot of the fundamentals of good science: understanding how data is collected, and accepting that the knowledge we seek is only accessible through approximation and proxy.
What I find very interesting is how well many people who are very likely not trained in working with any data is learning these things. It gives me a lot of hope that efforts to increase data literacy in people is an effective use of time, because it’s easily shown that many people can “get it” given the right conditions.
All thanks to awesome science communicators
There is a lot of panic and misinformation being posted about on the internet rumor mill right now. So a lot of what I’m pointing out today is actually the work of various experts in the medical field doing their best to counteract bad information with good information.
I foolishly didn’t keep track of all the tweets and people, so I’m borrowing a well-informed friend’s list of reputable science folk to follow on this issue: @HelenBranswell @angie_rasmussen @aetiology @marynmck @cmyeaton @mlipsitch @statnews.
Said friend probably wants to remain anonymous on my newsletter but they know who they are!
Bring us denominators!
One example is just the calculation of what is often quoted as “mortality rate”, which is roughly estimated to be somewhere around 1-3%, which puts the disease similar to the “Spanish Flu” of 1918. The number inherently depends on the denominator, and everyone admits that the total number of COVID-19 infections is an underestimate due to how mild symptoms can be.
That said, from my understanding on the topic, what people online have casually been calling the mortality rate is closer to case fatality rate, which is calculated as Deaths / Number of Confirmed Cases. Mortality rates (of the typical “1 death per 1000 population from cause X” sense) is too much of a lagging statistic. We won’t know if COVID-19 moves the needle on all-cause mortality until months later.
Interesting side note that some references indicate that The Great War in 1918 spurred censors to suppress news of the flu killing soldiers as early as 1916, and Spain just happened to not be in the war and thus didn’t suppress news of how people were dying of it to the same extent as other countries. The true origins of the flu strain is apparently still debated.
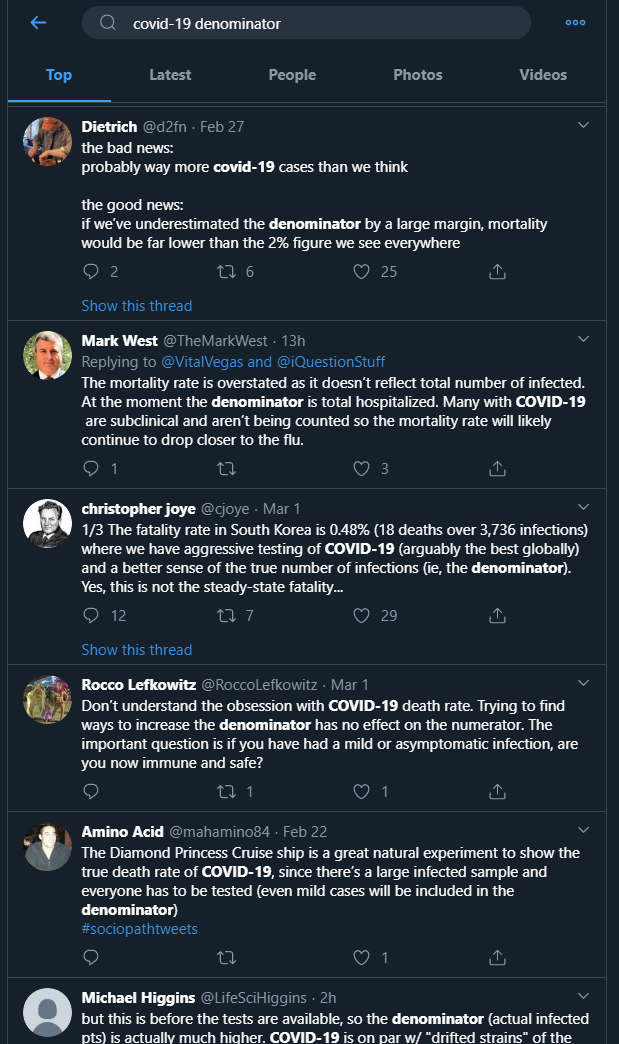
As you can see, a LOT of people, are interested in denominators all of a sudden, because it matters a lot right now whether the big scary number that implicates your future mortality is an overestimate or underestimate.
A LOT of data science work is about working with denominators and coming to an understanding of what the denominator of a ratio means before drawing conclusions. People are getting a first-hand look at just how messy that process is, as they struggle with the frustration of wanting to know “the true number” and being utterly unable to get it because of measurement/definition issues. In their minds, the real death rate exists out in platonic space, but it’s inaccessible due to measurement.
Looking at Distributions
As many of us know, averages hide a lot. This is obvious even to laypeople, especially when it is easy to hypothesize that certain segments of a population will react differently to the independent variable. We’re seeing this in discussions about vulnerable populations and the different fatality case rates, especially among the elderly and people with preexisting health conditions.

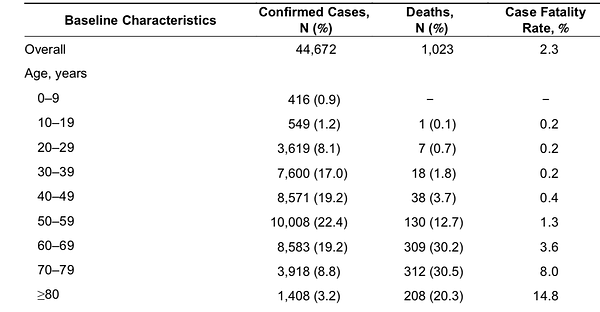

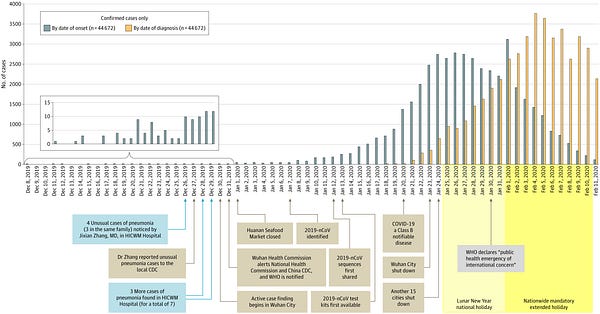
You can also see people start putting their understanding of what definitions go into the stats to use in interpreting official published stats.
Like in the above tweet about how cases below age 20 being low doesn’t mean the actual incidences of infections are low in that age group, but that there’s likely an interaction w/ the threshold for people being tested. And you could then draw the tentative conclusion that younger populations are less acutely affected by the diseases relative to older people.
Keep Teaching Data Literacy!
Data Science is communication, and I know it’s sometimes a drag to have to constantly teach various forms of data literacy as people come and go within an organization. It’s easy to feel like there’s a never-ending supply of people who seem hell-bent on doing shoddy data analysis to fit a chosen agenda. But take heart, most people can learn this stuff very quickly.