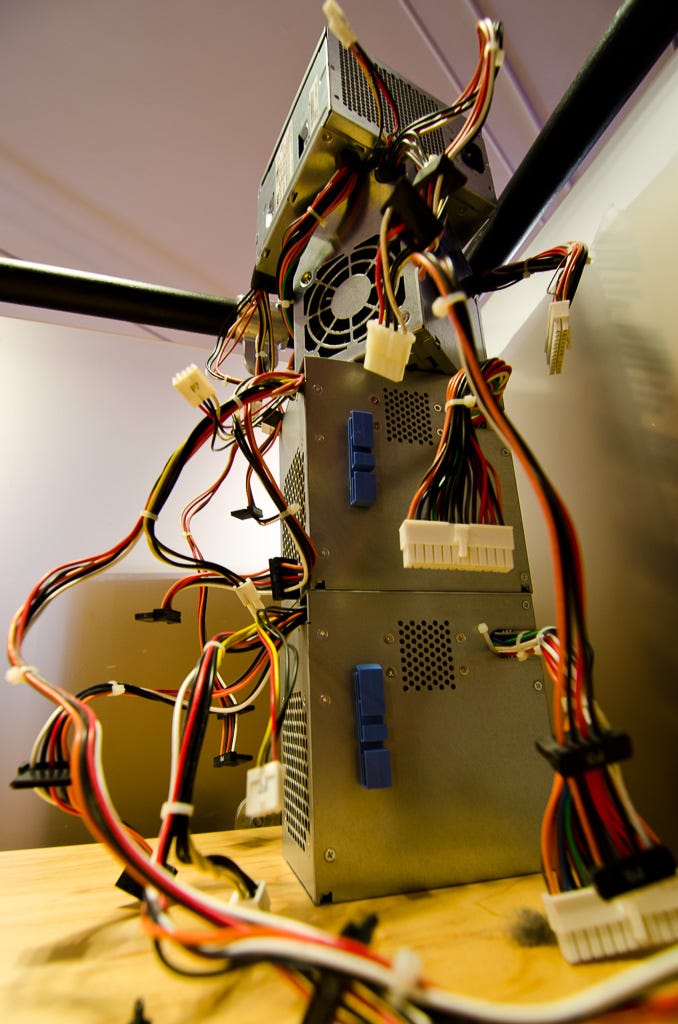
It’s election day in the US. Please vote if you’re able and haven’t. Then just take a breath and we’ll see what the remaining 27 decades of November 2020 hold for us together.
This week the childcare situation changed at home so the chaos-meter got cranked up an extra couple of notches. Add that to a recent deluge of high-visibility work that needed to be done and my brain was definitely not prepared for Friday to roll around the way it did. Also, as a further “Screw you!” to my brain and our continued pandemic-inspired lack of temporal sense, daylight savings is ending in the US this weekend. Hooray.
Being so distracted this week, I didn’t com across something on the internet that I wanted to write about, and thus asked the people if they had any questions. This one popped up that was quite interesting.

I think because data scientists are often seen to be “working on/with AI” (despite that not actually being true for the vast majority of us), we’re often seen as being on the most absolute bleeding edge of technology. This is of course made worse by the completely not representative (but highly visible and generally cool) data science people we see out in public.
Us mere data mortals aren’t like those highly visible individuals. Those people ARE working on the bleeding edge of tech, and have no choice but to use or invent new tools — it’s necessary for them to complete their work.
I get most of my daily work just fine using stats models from the 1800s (linear regression, Student’s t), and computer tech largely from the 60s (Unix, most algorithms, etc) and the 90s (Python). Probably the most modern tech advancement I take advantage of on a daily basis is my smartphone, which largely came together in the mid/late 2000s and lets me write articles like this from anywhere.
But the general question of tech adoption amongst data scientists is very interesting. There’s no one right answer, we all fall upon a very broad spectrum, from early adopters that use tons of the latest tech to people who will gladly use the same tooling for the next 20 years if they could.
Academic research into tech adoption is… useless here
The subject of technology adoption is a big topic in a bunch of academic fields. Here’s a brief 8-page paper from 2018 that summarizes a bunch of theories. It still is a hotbed of new research. Business-oriented fields want to understand it to influence it, sociological fields want to understand how and why societies adopt technology, psychological fields want to know how individuals decide to adopt tech.
But despite over 50 years of research and lots of interested parties, there’s still a ton of competing models and no one really knows why people choose to adopt the tech they do. A lot of it are variations on themes of “Hear about X, decide X is worth adopting somehow, adopt X”… (Not to fault the research, it’s really hard to understand what the heck is going on inside the brains of many humans”.
So I can’t lean on the literature to provide any overarching theoretical guidance. Instead, I’m going to borrow some of the ways of describing innovation adoption from the literature.
One of the earlier, and thus more well known, ways of describing phases of adoption is from Rodgers’ Diffusion of innovation from 1962. It’s talking about adoption of tech within broad society and breaks down adoption adopters into 5 phases of Innovators, Early adopters, Early majority, Late majority, and Laggards. It feels fairly reasonable, possibly because this language has been used to describe tech adoption for ages.
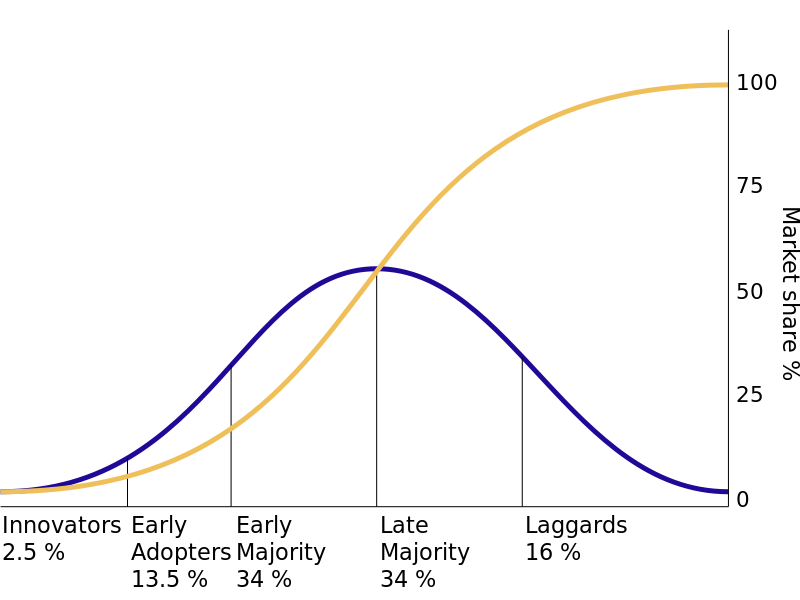
Behold! A Gaussian curve with…. weirdish arbitrary cutoff points, because sure, we’re just making models up anyways! Scanning the summary of the adopter categories on Wikipedia (because the original publication is a whole book I don’t have access to), it’s pretty surreal how the categories go from high social status for the Innovators all the way down to low social status for the Laggards. It’s a very tech-biased view of society. Plenty of that 1960’s… everything… going on.
While these terms are useful for talking about tech adoption in data science. We’re not exactly a complete “society” in sense the original theory was formulated. I’m also pretty skeptical if these proportions apply to the data science tooling environment.
The reason I believe the proportions are skewed non-normal is because in certain areas of data science, especially on the AI/ML side, the field has a LOT of researchers and academia generating tools for their own use right now. The pace of innovation is so high that mainstream industry hasn’t had time to digest anything before new stuff comes out. There should be a left skew right now because very few product even get into the 50% adoption mark before it’s overthrown by the next new thing. Most of us might actually wind up being “Early Majority” adopters and then the cool thing gets acquired.
Since the academic/historical tact I usually leverage to write isn’t working, we’ll switch gears and analyze the problem tool picking via reductionism instead.
For Orgs: Build, Buy, (but mostly just) Ignore
If you read about organizations adopting technology, the “Build or Buy” decision comes up constantly. (There’s also a separate major discussion thread about organizational tech adoption on employees resisting new tech, which I find very amusing in this discussion.) But the thing about the “Build or Buy” decision is that it presupposes the org is definitely going to adopt a technology.
Ignoring a new tech development is often not discussed when discussing tech decisions because it makes for boring articles about new tech (just… carry on. Done.). Why, of COURSE everyone wants new tech about $Foo
right? That’s why you clicked on the internet article!
But in reality, a lot of new awesome tech simply isn’t relevant to your current situation. Could a deep neural networks and machine vision be useful for your company’s accounts payable department? Maybe, if you’re very creative. But could you probably live without it? Yes. If anything, ignoring stuff is very much the default state of the world. In some contexts, it’s praised as “having strategic focus”.
The tech could also be relevant, but in such a state that you can’t make use of it. Maybe no on has the PhD to adapt the academic paper into product, or no one has the time to recreate and productionize a Java stack to use the latest tool that came out. There' are plenty of reasons why a company is going to decide they’re not going to bother working on using a new tech.
IF it’s decided that the investment is going to happen, then it boils down to a very pragmatic build or buy situation that’s written about in endless blog posts on the internet. It can be a pretty dry discussion of whether something
For individuals in an org: Ask who’s supporting it
As far as tech adoption goes in data science, I’m a fairly slow adopter. The biggest reason is because I work low in the stack as a UX Researcher, so the research tools and methodologies (logs analysis, surveys, metrics instrumentation, text coding, sampling, etc) are very well established. I’m also not being asked to solve new and unsolved methodological problems very often. Business questions often tread very similar ground over and over and over.
There’s some stuff ML techniques could help with here and there, but it’s not a hot problem space. So I don’t need to think about picking up new tools very often unless some SaaS vendor knocks on the door asking if I want to pay them money to have the privilege of re-implementing a data dictionary + product instrumentation to make “better” dashboards using an annoying “intuitive” web GUI.
I’m also busy enough with work and life that I don’t have time to plink around with code outside of work. Any minute spent fighting with a new tool to find a bug is wasted time. If the tool doesn’t pick up community backing and ultimately dies in the near future, that’s even worse.
Thanks to this highly skewed cost function, I don’t really adopt tools until the dust is well established. It’s like with VR gaming systems and home automation, I don’t need to spend hundreds on equipment that will go obsolete or have the vendor drop support after a couple of years. I’ll wait until some semblance of standards or longevity shows up because I don’t want to be left holding a bag desperately hacking fixes to keep my stuff running past the end of life date.
The same line of thinking could be said of any data pipeline solution that I slap together at work using whatever new tech I decide to install. If it becomes useful, someone’s going to have to maintain it when it breaks.
I don’t want to be that person. I especially don’t want to be that person if the new tech gets stale and I have to run a porting operation.
So in a work/production situation, the “build or buy” decision is bigger than you, even if you’re the only driver and direct consumer. It involves your whole team, potentially other teams, possibly the entire company. Your choices are much more constrained. It’s actually very freeing as an individual because it usually gives a very easy way to decide if something would be adopted or not.
Know those companies and the highly visible data scientists that work on the cutting edge stuff? They when through a very similar decision-making process too. They didn’t make a new thing because they felt like burning millions in headcount expense for the fun of it. They did the calculus and decided that having (or creating) the new tech is worth more than the entire total cost of ownership.
For individuals: but for learning/fun/etc
But let’s break free of organizational constraints and play with data science in our own time, either to learn more or just because we enjoy it. How should we pick new tools to use when stuff is constantly coming out?
First off, when in doubt, follow the crowd.
Not because I believe that there’s a lot of wisdom in the crowds when it comes to product decisions — tech fads are always coming and going regardless of technical merit (hi, blockchain!).
Instead, following the crowd is due to how community support and install base are important factors for the continued life of any tool. If Linux didn’t have tons of users and companies literally making millions and billions of dollars using and investing in it, it would’ve died. But because it has all that developer and industry support, to the tune of many billions of fake consultancy valuation points, Linux isn’t going anywhere soon.
That said, crowds have been wrong about products before. History is littered with the corpses of products that were “too early for its time” that later got revived by a different company and became a hit. Other times, the thing everyone does is suboptimal, like all the cheaply manufactured goods that come out of Asia in the past few decades, but it’s good enough.
Crowds will offer you a weak promise of support, potential longevity, and the benefit that if everything goes horribly wrong, someone else has the exact same problem as you and might write about a migration plan.
Next, look for the gap between what a tool promises and what it does
Web pages about data tools are horrible as a rule. It’s very difficult to write approachable, easy to understand copy that can fully describe what a tool is supposed to do and how it fits within existing infrastructure. On top of that, SaaS marketing folk edit the copy and suddenly it’s promising the moon and suddenly has stock photos, with blue numbers.
Every tool always promises that things will be super easy and magical, even open source ones with no marketing function. They’ll sometimes attempt to wow you with curated screenshots or a demo server. The people who wrote the tool will always feel it’s the most intuitive thing on the planet, because it matches their brains perfectly. I can confidently say without close examination, that you do not possess the same brain as the creators. That would be creepy.
If you don’t believe me, go and try to learn Blender without watching a few hours of YouTube tutorials. For those who don’t know much about 3d rendering software, Blender’s a super powerful open source tool that has a notoriously difficult to learn UI relative to various industry-standard 3d modeling programs.
Roll the dice, there might be no right answer
Once you hit the “I think I can use this new tool to do something”, there’s nothing left to do but to just give it a spin. Just remember that you could be completely wrong and may need to abort. That’s okay!
More importantly, realize that there might not be a “correct” tool to use right now. The one that might be the industry leader 5 years in the future may not exist yet. The successful tool you pick and love might get bought out by a big megacorp and slowly strangled to death. It’s a crazy world out there. You can’t guard against all this stuff.
Finally, always be ready to jump ship
For all the reasons stated above, you don’t want to commit entirely to a tech that is clouded in uncertainty. At the organizational level, it’s often called vendor lock-in in worrisome tones.
There’s always going to be a certain degree of integration and lock-in when two systems interface, but short of resting your entire software foundation upon a single vendor’s proprietary systems, the risk can be mitigated somewhat.
You can always try to be prepared to jump to an alternative, which means keeping abreast of competing tools in the same space. Usually there’s a 2nd-place product (or a tied-for-first) that works similarly enough that you don’t have to do a giant architectural re-shuffle to get things working again. You’ll still wind up throwing out tons of obsoleted code, but the design and algorithms should largely be the same…
Hopefully.
If you’re working with more specialized software that has no real competitor, you don’t need me to tell you how much more complex and unique that situation is, so I’ll skip over that wrinkle.
I hope you see why in my laziness I choose to be slow on the adoption side to avoid thinking too much about all these risks.
About this newsletter
I’m Randy Au, currently a quantitative UX researcher, former data analyst, and general-purpose data and tech nerd. The Counting Stuff newsletter is a weekly data/tech blog about the less-than-sexy aspects about data science, UX research and tech. With occasional excursions into other fun topics.
Comments and questions are always welcome, they often give me inspiration for new posts. Tweet me. Always feel free to share these free newsletter posts with others.
All photos/drawings used are taken/created by Randy unless otherwise noted.