This week I’ll do something that I don’t really do too often, look at academic paper! This is PRIMARILY because I don’t participate in any academic communities and don’t have much mental bandwidth to monitor papers. So if you, kind reader, ever spot a fun paper in the wild (on just about any subject), ping me! Y’all know I get excited at all sorts of rabbit holes.
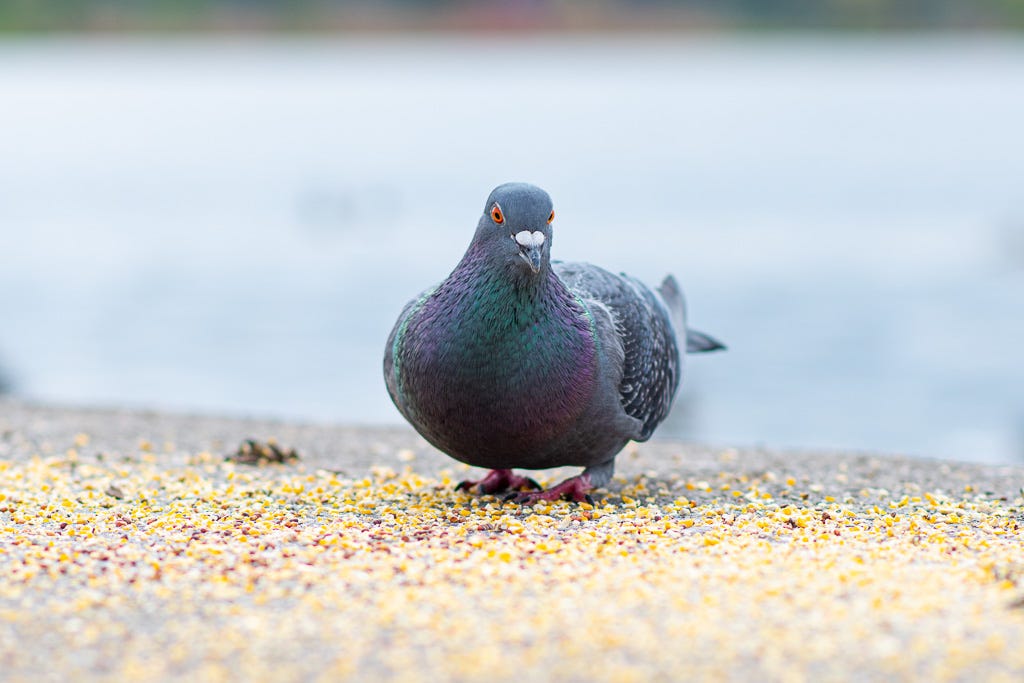
A month ago, Stuart Buck pinged me a couple of papers related to data cleaning and other aspects about data analysis/epistemology. (Many thanks!!) Since time is always in short supply, I picked the most fun one to cover today.
“The Influence of Hidden Researcher Decisions in Applied Microeconomics” by Nick Huntington-Klein et al. (2020, PDF available from here).
As a data analyst to my core, I can’t help being fascinated by “many analysts on same problem/data set” type research. Get a bunch of experienced data practitioners, have them all work on the same data set, see to what extent their results agree or differ from each other. It’s a methodology that exposes a lot of uncomfortable features about how data analysis, and science itself, is done.
This methodology is apparently a pretty new development? Today’s paper said that there was a paper using the method in 2018, and outside of academia there had been a notable example 2016.
As for why I found this particular paper interesting, and why Stuart brought it to my attention, here’s the business end of the abstract, my own emphasis added:
We find large differences in data preparation and analysis decisions, many of which would not likely be reported in a publication. No two replicators reported the same sample size. Statistical significance varied across replications, and for one of the studies the effect's sign varied as well. The standard deviation of estimates across replications was 3-4 times the typical reported standard error.
Hold up, varied in SIGN?!
Yes, meaning one of the people using the same data set and given the same research question and some constraints, wound up finding the opposite effect as everyone else.
That scares me to the very core of my being. It means that my own work, and the work of perhaps everyone else on the planet, might have accidentally stumbled and found an opposing significant effect simply by making various tweaks to how the data was formalized, coded, and cleaned.
Hopefully, for the type of work that I do in industry, things like triangulation from other signal sources can act as guard rails. Sure, my analysis might have spurred a decision that goes in the opposite direction, but we’d notice quickly that other stuff was tanking and we’d be able to back off.
Okay, so let’s get into it
The authors of this paper noticed that previous attempts at using multiple analysts on a single problem all gave researchers had given all the analysts data that was in a somewhat usable state. Since the “degrees of freedom” available to the analyst is one of the main drivers of differences between analyst results, that leaves a giant area of analysis out — data cleaning!
As we all know, there are LOTS of decisions to be made when cleaning up data — especially funky, messy data that had been generated and reported by governments over decades of time. Little decisions like how to handle missing entries, how every single concept cutoff and operationalization is defined, very often don’t make it into the final paper. Even if some of those details make it into a paper, readers and reviewers might skim over the details since there’s so many and seem trivial. This was the place the authors wanted to examine at.
Obviously, if you want to see how cleaning data affects things, you need to give them 1) data that’s dirty enough to warrant cleaning, 2) is available to lots of people, and 3) a task that’s clear enough that everyone is headed in the same direction. They turned to looking for papers published in respected economics journals (the authors are economists and thus interested in economic research methods) that used openly available data (such as the US Census).
So the general flow of this project is going to be :
Find some papers that use public data and yield a single estimate
Find qualified people to try to replicate the papers
Have them do a simplified replication of the studies
See what the replicators did
That tasks — Papers and data
Candidate papers also had have a single causal estimate of interest that can be replicated, not be too famous of a result that participants would recognize it from replication instructions, don’t rely on too much domain knowledge or methods (or at least they must be easily explained to participants).
Two papers were found from different subfields that fit the criteria. The authors go to pretty big lengths to detail what their decision process and reasoning was for each step of the whole experiment.
Their first paper is Black et al. (2008). Staying in the Classroom and Out of the Maternity Ward? The Effect of Compulsory Schooling Laws on Teenage Births. This paper tries to answer if mandatory school ages had an effect on teenage childbirth. It originally uses data from the US and Norway, as well as trying to figure out if the effect is due to improving human capital (e.g. improving the situation of the students) or through the “incarceration effect” (e.g. it’s harder to get pregnant if you’re locked in a shared environment 8 hours a day). This task used US Census data plus a provided Word file of the compulsory school age for each state at various points in time.
The second paper is Fairlie et al. (2011). Is Employer-based Health Insurance a Barrier to Entrepreneurship? This one hypothesizes that access to health insurance allows people more freedom to become entrepreneurs. Since the main source of health insurance is from employment until people get access to Medicare at age 65, the original authors compared people aged 64 years, 11 months vs people 65 years old to see what effect it has on people starting businesses. This task used the Current Population Survey data from the Census bureau.
The authors specifically call out that their goal is not to exactly replicate either paper in question, so this allowed them to include instructions to their replicator participants that specifically tell them to do certain things in order to simplify the process. This includes telling them to focus on specific date ranges or age groups to avoid extra complications, giving operationalized definitions like “teenage pregnancy as having a child by age 18”, or to only look at subjects who turned from 64 to 65 within the 4-month data collection period of the survey data used.
On top of all this, the instructions also try to make sure the replicators are approaching everything from similar positions. They’re told the basic research question and to analyze as if they were writing for their own paper publication. They also can use any statistics package they want, and use assistants if that’s how they normally do things. They’re supposed to show “how you would estimate this effect, if you’d had this question, this idea for identification, and had chosen this particular sample.”
In the end, replicators are asked to come up with just one “headline” estimation, and weren’t told to do alternate analyses, check for robustness etc. The headline was the one estimate that they’d put into the abstract of the paper if they were publishing. The replicators can (and did) do alternate analysis if they want, but the headline was the one deemed most important for comparison purposes.
The initial instructions gave replicators 7 months to complete the task, but the deadline was pushed back an extra 2 months later for a total of 9 months to do the work.
Participants
Since this is an economics paper looking for replications of economics research, obviously they wanted practicing economics researchers to be doing the replications. Not random undergrad students, no random data scientists from the internet, no riffraff like me.
Who qualifies? Essentially, someone who is actively publishing to applied economics research journals.
They recruited by asking department heads of respected econ departments to forward a recruitment email to faculty. They also recruited via Twitter. Interesting bit is that while both routes did ultimately give them usable participants, Twitter wound up giving more.
They ultimately had 51 qualified researchers who signed up to complete at least one replication, 37 came from Twitter and 14 from the emails to departments. By the end of the study, 12 had completed at least one replication, 10 completing a single replication tasks while 2 completed both tasks.
Tasks were assigned based on field of interest and whether they wanted to do one or both replications. For people who wanted to do only one, if the researcher worked in a field that was relevant to a task, they got assigned the relevant task. People with relevant experience in both, or neither task were randomly assigned. People who wanted to do both replications were assigned their first task in this way.
So what happened?
Out of the 14 replications completed, they wound up with 7 replications for each task.
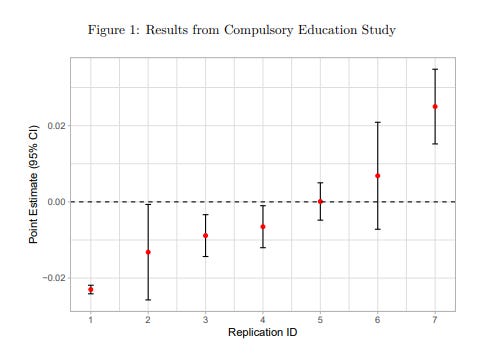
The replication about compulsory education’s effect on teenage pregnancy had the surprising result that one group wound up finding a significant effect of opposite sign.
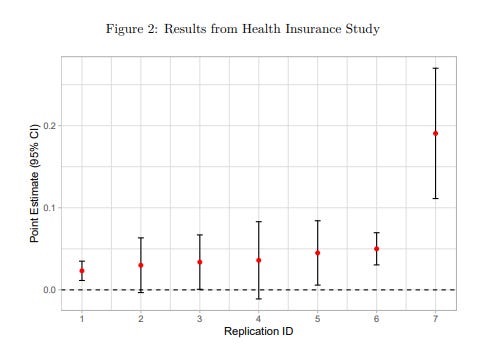
Meanwhile, the replication about whether getting access to healthcare (Medicare) would affect entrepreneurship mostly had replications in the same range, which looks encouraging. Then there’s one replicator that comes up with an massively different estimate.
So does the paper go into how these differences came to be?
Yes, and it probably comprises a bit under half the paper’s length. All participants were asked to send their code and data over so that the authors can replicate everyone’s work. The authors say this was done and they could reproduce all the estimates.
First, at the Compulsory schooling/teen pregnancy replication task
For this task, everyone did NOT use any grad students/assistants to help out, and everyone used Stata. Everyone had to get data from the US Census and clean the data for use.
The instructions said that women with first births age 14 or below should be dropped. However, one study instead dropped those with first births age 13 or below. Another kept these women but coded them as not being teenage pregnancies. One study did not limit the data to women aged 20-30, as instructed. One study did not match policy dates to individuals in the exact way described in the instructions. Afterwards, one replicator reported having made these decisions because they misread the instructions. Other cases may be due to thinking the differing decisions were more appropriate . — pp.15
So the instructions themselves occasional had issues from mistakes or misinterpretation. But there were also places that replicators needed to make decisions because there weren’t explicit instructions, like the exact parameters to construct their analysis sample. Mistakes in interpretation led to some pretty huge differences.
These sample construction decisions led to different sample sizes from every replicator. No two replications had the same sample size, although most are similar. The smallest sample size is 831,139, driven by dropping women without children and one of the census samples. The largest is 4,271,245, driven by including women outside the age range of 20-30. All other samples are fairly similar but not exactly the same, ranging from 1.64 million to 1.70 million. — pp.15
The authors then decided to do something pretty interesting. Note that there’s 2 chunks of decisions being made be researchers, 1) all the decisions used to build the sample, 2) all the decisions in the analysis model to estimate the effects. It’s not clear how much each chunk is contributing to the differences. So they take all the samples and run the same basic model on all of them.
This reduces differences between estimates, suggesting that some of the differences are due to analysis rather than sample construction. But important differences remain, and the sign is still not consistent. Of particular interest are replications 1 and 7, which do not differ in sample construction in any obvious way, and which likely would have reported identical data construction procedures if these were real studies, but for which sample sizes differ by about 4,000. When using identical models they have similarly-sized estimates of opposite signs. — pp.16 [emphasis added]
When it came to looking at the analysis decisions, it seemed everyone chose to use very similar choices because the problem resembled a typical difference-in-difference experimental design, so everyone used regression with two-way fixed effects.
Despite a binary dependent variable, all seven used ordinary least squares rather than logit or some other nonlinear model. — pp.18, this sentence made me smile.
The authors then noted that “because all studies used the same design, differences in point estimates can only be driven by differences in data construction or the choice of controls or regression command.” So they arbitrarily picked one replication’s data set and ran everyone’s analysis on that one data set, the differences remained the same between models. That meant it wasn’t the regression command, nor the data construction, meaning choice of control variables was causing the differences between models.
Next the Health Insurance/Entrepreneurship replication
The story here follows a similar pattern, people did some stuff while cleaning and building their data set, and also did some other stuff while making their models. The end result wound up being much more similar, but there were still interesting parts.
This cohort of replicators had three of the seven replicators using graduate student assistances doing at least some of the work. There was also one R user! The rest use Stata.
As with before, the building of samples saw lots of differences between replicators. For example, despite the instructions given with the task, four of the replicators expanded age ranges examined. Two later said they misunderstood the instructions, two said they thought their choice was more appropriate.
Just like with the other task, everyone had different sample sizes.
When the authors ran a single basic model on all the sample sizes (while restricting the expanded-range age groups a bit because those needed age control variables), the results came out to be fairly similar, suggesting that the models were what led to the observed differences.
The authors note that the analysis prompt and data lends itself to a “regression discontinuity design (RDD)”. They noted that “Some replicators explicitly used regression discontinuity, while others compared the raw average above and below the cutoff, in effect a regression discontinuity with a zero-order polynomial.”
But within the design, all the replicators made lots of difference choices. Some used nonlinear models, some used linear ones. Control variables and their definitions varied massively.
The authors also did the “run all analyses on the same arbitrarily picked piece of data” analysis to isolate where the variation comes from. They ran into issues because the models that used the expanded age ranges, they hade to make some changes to the models (dropping polynomial terms for age) and it broke some of the model code. From what they managed to get running, things seemed to suggest that yes, the models appeared to be the source of variation.
So what did I take away from this paper?
That the truth we “find” while doing our work day to day, whether it’s the big “Truth” of science, or the little “truth” of quick and dirty analytics, sit on a surprisingly shaky foundation.
Any choice that we make, or have made for us, will put our work on a path that leads to different final results. There’s no truly perfect equivalent. Sometimes the different is small, and sometimes they’re even the wrong sign.
These data analysis issues aren’t new. They’ve existed since pre-history when humans made observations and came to conclusions about it. Even the concept of statistical significance plainly states that there is a nonzero chance that we’ve actually accepted a false positive alternate hypothesis. Out of the countless alternative hypotheses accepted over the years, there’s no way to know exactly how many have been false positives.
And yet, society, science, everything has progressed to where we are today. On average, we’ve managed to find and keep true things, while slowly reducing the number of things we falsely accepted. It’s a sketchy, lossy, dodgy system. We probably also have accidentally disproven true things and will have to rediscover them again in the future.
My belief is that we’ve managed as a species to successfully keep acquiring new truths is because the world continues to exist outside of us. Even if a thousand analysts make a thousand mistakes stating truths about the world, it will keep presenting counter-evidence to anyone looking until we eventually get it right.
Verification. Replication. Triangulation.
We don’t do any of those things enough, not in Academia, and certainly not in industry. The incentives and time constraints rarely let us. Even though we live in a swirling pit of epistemic uncertainty.
Could it be better? Obviously. We see evidence of this in the various forms of replication issues that is popping up in various fields of science. While there’s lots of debate about what needs to be done about it, I have faith that on average, over time, we get better at this.
About this newsletter
I’m Randy Au, currently a Quantitative UX researcher, former data analyst, and general-purpose data and tech nerd. The Counting Stuff newsletter is a weekly data/tech blog about the less-than-sexy aspects about data science, UX research and tech. With occasional excursions into other fun topics.
All photos/drawings used are taken/created by Randy unless otherwise noted.
Supporting this newsletter:
This newsletter is free, share it with your friends without guilt! But if you like the content and want to send some love, here’s some options:
Tweet me - Comments and questions are always welcome, they often inspire new posts